GoSecure Blog
Microsoft SharePoint Emergency RCE Patches
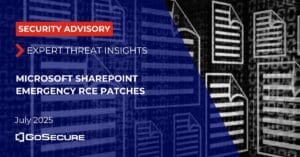
Threat Hunt of the Month: External Remote Services Exploited for Initial Access and Persistence
In March 2025, GoSecure Threat Hunters investigated a growing threat targeting external remote services—such as VPN access points, Remote Desktop Protocol (RDP), and firewall management interfaces—frequently exposed to the public internet. Recent activity has revealed threat actors exploiting Fortinet vulnerabilities (CVE-2024-55591 and CVE-2025-24472) to gain unauthorized access and create persistent admin accounts through automation scripts. These tactics mirror methods used by ransomware groups like Play and LAPSUS$, who rely on stolen credentials and unprotected remote services to infiltrate corporate networks.
Identifying and Investigating Suspicious Outbound TLD Traffic
Cybercriminals are constantly finding new ways to bypass traditional security measures, and one of their latest tactics involves using obscure Top-Level Domains (TLDs) to facilitate malicious activities. From data exfiltration and phishing to command-and-control (C2) operations, these domains provide attackers with an easy way to evade detection.
Oracle Cloud Breach: A Strategic Response to an Identity-Layer Threat
On March 21, 2025, security researchers identified a threat actor, operating under the alias rose87168, attempting to sell over six million records allegedly exfiltrated from Oracle Cloud’s Single Sign-On (SSO) and LDAP services. This breach is suspected to stem from a vulnerability within the login infrastructure of login.(region-name).oraclecloud.com.
Talk To Your Malware – Integrating AI Capability in an Open-Source C2 Agent
TL;DR
Introducing malware (implants) that uses AI to generate and execute code. Operators can now simply “talk” to their implants, and it will magically execute the requested instructions. For example, an operator could say: “Scan every user’s home folder and pack any office file under 2MB inside a single archive saved in C:/test/output.zip“, and the implant would obey.
This has several advantages: